-
Cybercrime Resilience in the Era of Advanced Technologies: Evidence from the Financial Sector of a Developing Country
-
A Literature Review on Security in the Internet of Things: Identifying and Analysing Critical Categories
-
Machine Learning and Deep Learning Paradigms: From Techniques to Practical Applications and Research Frontiers
Journal Description
Computers
Computers
is an international, scientific, peer-reviewed, open access journal of computer science, including computer and network architecture and computer–human interaction as its main foci, published monthly online by MDPI.
- Open Access— free for readers, with article processing charges (APC) paid by authors or their institutions.
- High Visibility: indexed within Scopus, ESCI (Web of Science), dblp, Inspec, Ei Compendex, and other databases.
- Journal Rank: JCR - Q2 (Computer Science, Interdisciplinary Applications) / CiteScore - Q2 (Computer Networks and Communications)
- Rapid Publication: manuscripts are peer-reviewed and a first decision is provided to authors approximately 15.5 days after submission; acceptance to publication is undertaken in 3.8 days (median values for papers published in this journal in the second half of 2024).
- Recognition of Reviewers: reviewers who provide timely, thorough peer-review reports receive vouchers entitling them to a discount on the APC of their next publication in any MDPI journal, in appreciation of the work done.
Impact Factor:
2.6 (2023);
5-Year Impact Factor:
2.4 (2023)
Latest Articles
A Novel Autonomous Robotic Vehicle-Based System for Real-Time Production and Safety Control in Industrial Environments
Computers 2025, 14(5), 188; https://doi.org/10.3390/computers14050188 - 12 May 2025
Abstract
Industry 4.0 has revolutionized the way companies manufacture, improve, and distribute their products through the use of new technologies, such as artificial intelligence, robotics, and machine learning. Autonomous Mobile Robots (AMRs), especially, have gained a lot of attention, supporting workers with daily industrial
[...] Read more.
Industry 4.0 has revolutionized the way companies manufacture, improve, and distribute their products through the use of new technologies, such as artificial intelligence, robotics, and machine learning. Autonomous Mobile Robots (AMRs), especially, have gained a lot of attention, supporting workers with daily industrial tasks and boosting overall performance by delivering vital information about the status of the production line. To this end, this work presents the novel Q-CONPASS system that aims to introduce AMRs in production lines with the ultimate goal of gathering important information that can assist in production and safety control. More specifically, the Q-CONPASS system is based on an AMR equipped with a plethora of machine learning algorithms that enable the vehicle to safely navigate in a dynamic industrial environment, avoiding humans, moving machines, and stationary objects while performing important tasks. These tasks include the identification of the following: (i) missing objects during product packaging and (ii) extreme skeletal poses of workers that can lead to musculoskeletal disorders. Finally, the Q-CONPASS system was validated in a real-life environment (i.e., the lift manufacturing industry), showcasing the importance of collecting and processing data in real-time to boost productivity and improve the well-being of workers.
Full article
Open AccessArticle
Interpretable Deep Learning for Diabetic Retinopathy: A Comparative Study of CNN, ViT, and Hybrid Architectures
by
Weijie Zhang, Veronika Belcheva and Tatiana Ermakova
Computers 2025, 14(5), 187; https://doi.org/10.3390/computers14050187 - 12 May 2025
Abstract
Diabetic retinopathy (DR) is a leading cause of vision impairment worldwide, requiring early detection for effective treatment. Deep learning models have been widely used for automated DR classification, with Convolutional Neural Networks (CNNs) being the most established approach. Recently, Vision Transformers (ViTs) have
[...] Read more.
Diabetic retinopathy (DR) is a leading cause of vision impairment worldwide, requiring early detection for effective treatment. Deep learning models have been widely used for automated DR classification, with Convolutional Neural Networks (CNNs) being the most established approach. Recently, Vision Transformers (ViTs) have shown promise, but a direct comparison of their performance and interpretability remains limited. Additionally, hybrid models that combine CNN and transformer-based architectures have not been extensively studied. This work systematically evaluates CNNs (ResNet-50), ViTs (Vision Transformer and SwinV2-Tiny), and hybrid models (Convolutional Vision Transformer, LeViT-256, and CvT-13) on DR classification using publicly available retinal image datasets. The models are assessed based on classification accuracy and interpretability, applying Grad-CAM and Attention-Rollout to analyze decision-making patterns. Results indicate that hybrid models outperform both standalone CNNs and ViTs, achieving a better balance between local feature extraction and global context awareness. The best-performing model (CvT-13) achieved a Quadratic Weighted Kappa (QWK) score of 0.84 and an AUC of 0.93 on the test set. Interpretability analysis shows that CNNs focus on fine-grained lesion details, while ViTs exhibit broader but less localized attention. These findings provide valuable insights for optimizing deep learning models in medical imaging, supporting the development of clinically viable AI-driven DR screening systems.
Full article
(This article belongs to the Special Issue Application of Artificial Intelligence and Modeling Frameworks in Health Informatics and Related Fields)
►▼
Show Figures
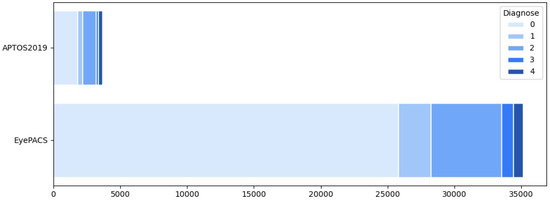
Figure 1
Open AccessArticle
Test-Time Training with Adaptive Memory for Traffic Accident Severity Prediction
by
Duo Peng and Weiqi Yan
Computers 2025, 14(5), 186; https://doi.org/10.3390/computers14050186 - 10 May 2025
Abstract
Traffic accident prediction is essential for improving road safety and optimizing intelligent transportation systems. However, deep learning models often struggle with distribution shifts and class imbalance, leading to degraded performance in real-world applications. While distribution shift is a common challenge in machine learning,
[...] Read more.
Traffic accident prediction is essential for improving road safety and optimizing intelligent transportation systems. However, deep learning models often struggle with distribution shifts and class imbalance, leading to degraded performance in real-world applications. While distribution shift is a common challenge in machine learning, Transformer-based models—despite their ability to capture long-term dependencies—often lack mechanisms for dynamic adaptation during inferencing. In this paper, we propose a TTT-Enhanced Transformer that incorporates Test-Time Training (TTT), enabling the model to refine its parameters during inferencing through a self-supervised auxiliary task. To further boost performance, an Adaptive Memory Layer (AML), a Feature Pyramid Network (FPN), Class-Balanced Attention (CBA), and Focal Loss are integrated to address multi-scale, long-term, and imbalance-related challenges. Our experimental results show that our model achieved an overall accuracy of 96.86% and a severe accident recall of 95.8%, outperforming the strongest Transformer baseline by 5.65% in accuracy and 9.6% in recall. The results of our confusion matrix and ROC analyses confirm our model’s superior classification balance and discriminatory power. These findings highlight the potential of our approach in enhancing real-time adaptability and robustness under shifting data distributions and class imbalances in intelligent transportation systems.
Full article
(This article belongs to the Special Issue AI in Its Ecosystem)
►▼
Show Figures
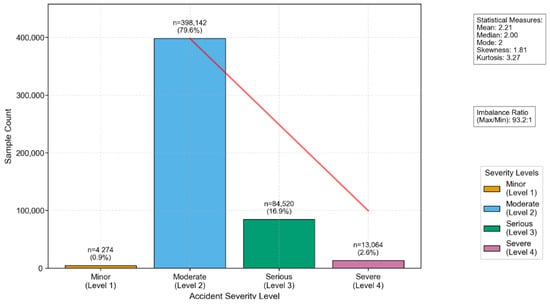
Figure 1
Open AccessSystematic Review
The Influence of Artificial Intelligence Tools on Learning Outcomes in Computer Programming: A Systematic Review and Meta-Analysis
by
Manal Alanazi, Ben Soh, Halima Samra and Alice Li
Computers 2025, 14(5), 185; https://doi.org/10.3390/computers14050185 - 9 May 2025
Abstract
This systematic review and meta-analysis investigates the impact of artificial intelligence (AI) tools, including ChatGPT 3.5 and GitHub Copilot, on learning outcomes in computer programming courses. A total of 35 controlled studies published between 2020 and 2024 were analysed to assess the effectiveness
[...] Read more.
This systematic review and meta-analysis investigates the impact of artificial intelligence (AI) tools, including ChatGPT 3.5 and GitHub Copilot, on learning outcomes in computer programming courses. A total of 35 controlled studies published between 2020 and 2024 were analysed to assess the effectiveness of AI-assisted learning. The results indicate that students using AI tools outperformed those without such aids. The meta-analysis findings revealed that AI-assisted learning significantly reduced task completion time (SMD = −0.69, 95% CI [−2.13, −0.74], I2 = 95%, p = 0.34) and improved student performance scores (SMD = 0.86, 95% CI [0.36, 1.37], p = 0.0008, I2 = 54%). However, AI tools did not provide a statistically significant advantage in learning success or ease of understanding (SMD = 0.16, 95% CI [−0.23, 0.55], p = 0.41, I2 = 55%), with sensitivity analysis suggesting result variability. Student perceptions of AI tools were overwhelmingly positive, with a pooled estimate of 1.0 (95% CI [0.92, 1.00], I2 = 0%). While AI tools enhance computer programming proficiency and efficiency, their effectiveness depends on factors such as tool functionality and course design. To maximise benefits and mitigate over-reliance, tailored pedagogical strategies are essential. This study underscores the transformative role of AI in computer programming education and provides evidence-based insights for optimising AI-assisted learning.
Full article
(This article belongs to the Section Cloud Continuum and Enabled Applications)
►▼
Show Figures
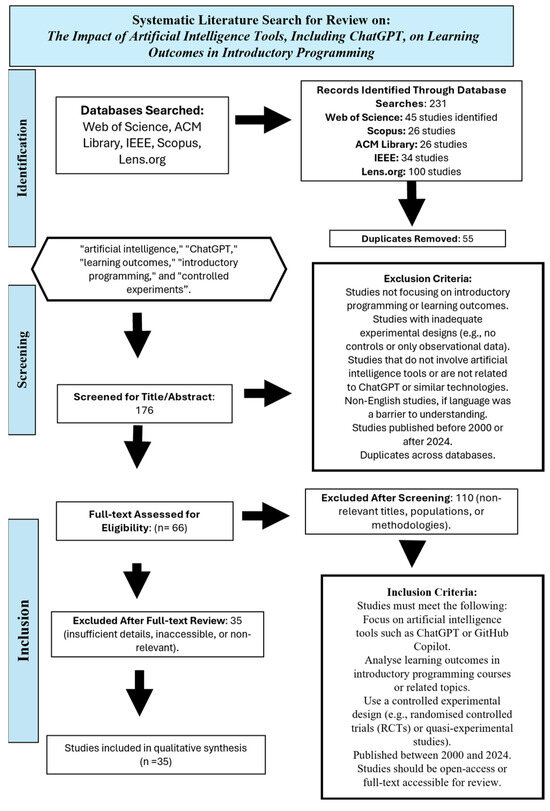
Figure 1
Open AccessArticle
Driver Distraction Detection in Extreme Conditions Using Kolmogorov–Arnold Networks
by
János Hollósi, Gábor Kovács, Mykola Sysyn, Dmytro Kurhan, Szabolcs Fischer and Viktor Nagy
Computers 2025, 14(5), 184; https://doi.org/10.3390/computers14050184 - 9 May 2025
Abstract
Driver distraction can have severe safety consequences, particularly in public transportation. This paper presents a novel approach for detecting bus driver actions, such as mobile phone usage and interactions with passengers, using Kolmogorov–Arnold networks (KANs). The adversarial FGSM attack method was applied to
[...] Read more.
Driver distraction can have severe safety consequences, particularly in public transportation. This paper presents a novel approach for detecting bus driver actions, such as mobile phone usage and interactions with passengers, using Kolmogorov–Arnold networks (KANs). The adversarial FGSM attack method was applied to assess the robustness of KANs in extreme driving conditions, like adverse weather, high-traffic situations, and bad visibility conditions. In this research, a custom dataset was used in collaboration with a partner company in the field of public transportation. This allows the efficiency of Kolmogorov–Arnold network solutions to be verified using real data. The results suggest that KANs can enhance driver distraction detection under challenging conditions, with improved resilience against adversarial attacks, particularly in low-complexity networks.
Full article
(This article belongs to the Special Issue Emerging Trends in Machine Learning and Artificial Intelligence)
►▼
Show Figures
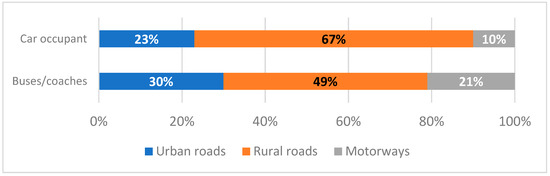
Figure 1
Open AccessArticle
Domain- and Language-Adaptable Natural Language Interface for Property Graphs
by
Ioannis Tsampos and Emmanouil Marakakis
Computers 2025, 14(5), 183; https://doi.org/10.3390/computers14050183 - 9 May 2025
Abstract
Despite the growing adoption of Property Graph Databases, like Neo4j, interacting with them remains difficult for non-technical users due to the reliance on formal query languages. Natural Language Interfaces (NLIs) address this by translating natural language (NL) into Cypher. However, existing solutions are
[...] Read more.
Despite the growing adoption of Property Graph Databases, like Neo4j, interacting with them remains difficult for non-technical users due to the reliance on formal query languages. Natural Language Interfaces (NLIs) address this by translating natural language (NL) into Cypher. However, existing solutions are typically limited to high-resource languages; are difficult to adapt to evolving domains with limited annotated data; and often depend on Machine Learning (ML) approaches, including Large Language Models (LLMs), that demand substantial computational resources and advanced expertise for training and maintenance. We address these limitations by introducing a novel dependency-based, training-free, schema-agnostic Natural Language Interface (NLI) that converts NL queries into Cypher for querying Property Graphs. Our system employs a modular pipeline-integrating entity and relationship extraction, Named Entity Recognition (NER), semantic mapping, triple creation via syntactic dependencies, and validation against an automatically extracted Schema Graph. The distinctive feature of this approach is the reduction in candidate entity pairs using syntactic analysis and schema validation, eliminating the need for candidate query generation and ranking. The schema-agnostic design enables adaptation across domains and languages. Our system supports single- and multi-hop queries, conjunctions, comparisons, aggregations, and complex questions through an explainable process. Evaluations on real-world queries demonstrate reliable translation results.
Full article
(This article belongs to the Special Issue Natural Language Processing (NLP) and Large Language Modelling)
►▼
Show Figures
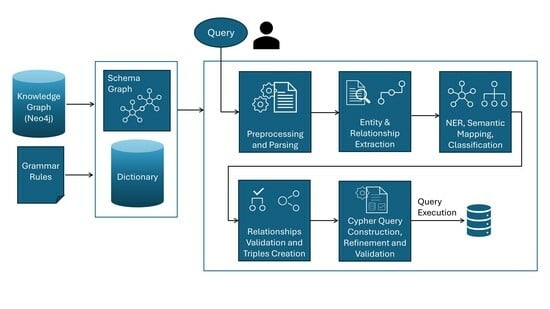
Graphical abstract
Open AccessArticle
AFQSeg: An Adaptive Feature Quantization Network for Instance-Level Surface Crack Segmentation
by
Shaoliang Fang, Lu Lu, Zhu Lin, Zhanyu Yang and Shaosheng Wang
Computers 2025, 14(5), 182; https://doi.org/10.3390/computers14050182 - 9 May 2025
Abstract
Concrete surface crack detection plays a crucial role in infrastructure maintenance and safety. Deep learning-based methods have shown great potential in this task. However, under real-world conditions such as poor image quality, environmental interference, and complex crack patterns, existing models still face challenges
[...] Read more.
Concrete surface crack detection plays a crucial role in infrastructure maintenance and safety. Deep learning-based methods have shown great potential in this task. However, under real-world conditions such as poor image quality, environmental interference, and complex crack patterns, existing models still face challenges in detecting fine cracks and often rely on large training parameters, limiting their practicality in complex environments. To address these issues, this paper proposes a crack detection model based on adaptive feature quantization, which primarily consists of a maximum soft pooling module, an adaptive crack feature quantization module, and a trainable crack post-processing module. Specifically, the maximum soft pooling module improves the continuity and integrity of detected cracks. The adaptive crack feature quantization module enhances the contrast between cracks and background features and strengthens the model’s focus on critical regions through spatial feature fusion. The trainable crack post-processing module incorporates edge-guided post-processing algorithms to correct false predictions and refine segmentation results. Experiments conducted on the Crack500 Road Crack Dataset show that, the proposed model achieves notable improvements in detection accuracy and efficiency, with an average F1-score improvement of 2.81% and a precision gain of 2.20% over the baseline methods. In addition, the model significantly reduces computational cost, achieving a 78.5–88.7% reduction in parameter size and up to 96.8% improvement in inference speed, making it more efficient and deployable for real-world crack detection applications.
Full article
(This article belongs to the Special Issue Machine Learning Applications in Pattern Recognition)
►▼
Show Figures
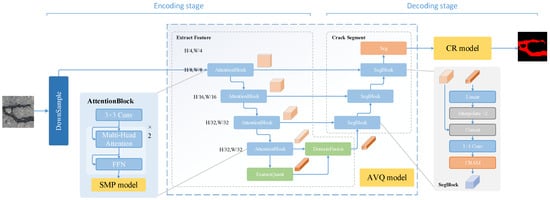
Figure 1
Open AccessArticle
Parallel Sort Implementation and Evaluation in a Dataflow-Based Polymorphic Computing Architecture
by
David Hentrich, Erdal Oruklu and Jafar Saniie
Computers 2025, 14(5), 181; https://doi.org/10.3390/computers14050181 - 7 May 2025
Abstract
This work presents two variants of an odd–even sort algorithm that are implemented in a dataflow-based polymorphic computing architecture. The two odd–even sort algorithms are the “fully unrolled” variant and the “compact” variant. They are used as test kernels to evaluate the polymorphic
[...] Read more.
This work presents two variants of an odd–even sort algorithm that are implemented in a dataflow-based polymorphic computing architecture. The two odd–even sort algorithms are the “fully unrolled” variant and the “compact” variant. They are used as test kernels to evaluate the polymorphic computing architecture. Incidentally, these two odd–even sort algorithm variants can be readily adapted to ASIC (Application-Specific Integrated Circuit) and FPGA (Field Programmable Gate Array) designs. Additionally, two methods of placing the sort algorithms’ instructions in different configurations of the polymorphic computing architecture to achieve performance gains are furnished: a genetic-algorithm-based instruction placement method and a deterministic instruction placement method. Finally, a comparative study of the odd–even sort algorithm in several configurations of the polymorphic computing architecture is presented. The results show that scaling up the number of processing cores in the polymorphic architecture to the maximum amount of instantaneously exploitable parallelism improves the speed of the sort algorithms. Additionally, the sort algorithms that were placed in the polymorphic computing architecture configurations by the genetic instruction placement algorithm generally performed better than when they were placed by the deterministic instruction placement algorithm.
Full article
(This article belongs to the Section Cloud Continuum and Enabled Applications)
►▼
Show Figures
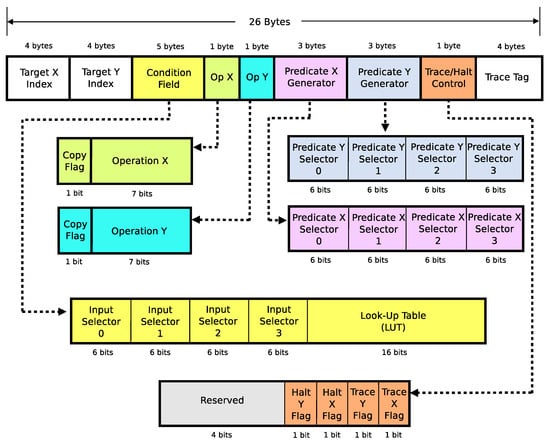
Figure 1
Open AccessArticle
Teachers’ Experiences with Flipped Classrooms in Senior Secondary Mathematics Instruction
by
Adebayo Akinyinka Omoniyi, Loyiso Currell Jita and Thuthukile Jita
Computers 2025, 14(5), 180; https://doi.org/10.3390/computers14050180 - 6 May 2025
Abstract
►▼
Show Figures
The quest for effective pedagogical practices in mathematics education has increasingly highlighted the flipped classroom model. This model has been shown to be particularly successful in higher education settings within developed countries, where resources and technological infrastructure are readily available. However, its implementation
[...] Read more.
The quest for effective pedagogical practices in mathematics education has increasingly highlighted the flipped classroom model. This model has been shown to be particularly successful in higher education settings within developed countries, where resources and technological infrastructure are readily available. However, its implementation in secondary education, especially in developing nations, has been a critical area of investigation. Building on our earlier research, which found that students rated the flipped classroom model positively, this mixed-method study explores teachers’ experiences with implementing the model for mathematics instruction at the senior secondary level. Since teachers play a pivotal role as facilitators of this pedagogical approach, their understanding and perceptions of it can significantly impact its effectiveness. To gather insights into teachers’ experiences, this study employs both close-ended questionnaires and semi-structured interviews. A quantitative analysis of participants’ responses to the questionnaires, including mean scores, standard deviations and Kruskal–Wallis H tests, reveals that teachers generally record positive experiences teaching senior secondary mathematics through flipped classrooms, although there are notable differences in their experiences. A thematic analysis of qualitative interview responses highlights the specific support systems essential for teachers’ successful adoption of the flipped classroom model in senior secondary mathematics instruction.
Full article
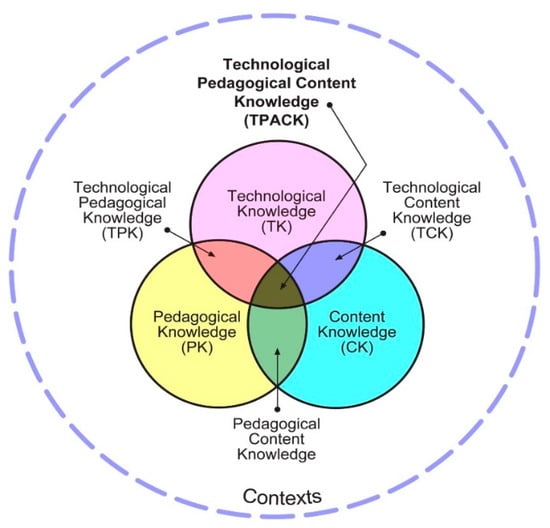
Figure 1
Open AccessArticle
Property-Based Testing for Cybersecurity: Towards Automated Validation of Security Protocols
by
Manuel J. C. S. Reis
Computers 2025, 14(5), 179; https://doi.org/10.3390/computers14050179 - 6 May 2025
Abstract
The validation of security protocols remains a complex and critical task in the cybersecurity landscape, often relying on labor-intensive testing or formal verification techniques with limited scalability. In this paper, we explore property-based testing (PBT) as a powerful yet underutilized methodology for the
[...] Read more.
The validation of security protocols remains a complex and critical task in the cybersecurity landscape, often relying on labor-intensive testing or formal verification techniques with limited scalability. In this paper, we explore property-based testing (PBT) as a powerful yet underutilized methodology for the automated validation of security protocols. PBT enables the generation of large and diverse input spaces guided by declarative properties, making it well-suited to uncover subtle vulnerabilities in protocol logic, state transitions, and access control flows. We introduce the principles of PBT and demonstrate its applicability through selected use cases involving authentication mechanisms, cryptographic APIs, and session protocols. We further discuss integration strategies with existing security pipelines and highlight key challenges such as property specification, oracle design, and scalability. Finally, we outline future research directions aimed at bridging the gap between PBT and formal methods, with the goal of advancing the automation and reliability of secure system development.
Full article
(This article belongs to the Special Issue Cyber Security and Privacy in IoT Era)
►▼
Show Figures
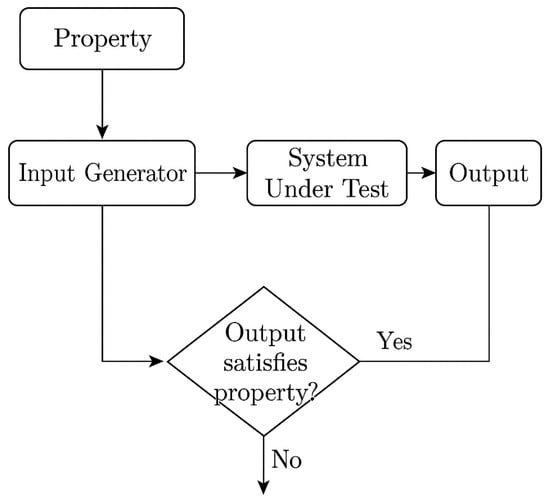
Figure 1
Open AccessArticle
A Hybrid Deep Learning Approach for Secure Biometric Authentication Using Fingerprint Data
by
Abdulrahman Hussian, Foud Murshed, Mohammed Nasser Alandoli and Ghalib Aljafari
Computers 2025, 14(5), 178; https://doi.org/10.3390/computers14050178 - 6 May 2025
Abstract
Despite significant advancements in fingerprint-based authentication, existing models still suffer from challenges such as high false acceptance and rejection rates, computational inefficiency, and vulnerability to spoofing attacks. Addressing these limitations is crucial for ensuring reliable biometric security in real-world applications, including law enforcement,
[...] Read more.
Despite significant advancements in fingerprint-based authentication, existing models still suffer from challenges such as high false acceptance and rejection rates, computational inefficiency, and vulnerability to spoofing attacks. Addressing these limitations is crucial for ensuring reliable biometric security in real-world applications, including law enforcement, financial transactions, and border security. This study proposes a hybrid deep learning approach that integrates Convolutional Neural Networks (CNNs) with Long Short-Term Memory (LSTM) networks to enhance fingerprint authentication accuracy and robustness. The CNN component efficiently extracts intricate fingerprint patterns, while the LSTM module captures sequential dependencies to refine feature representation. The proposed model achieves a classification accuracy of 99.42%, reducing the false acceptance rate (FAR) to 0.31% and the false rejection rate (FRR) to 0.27%, demonstrating a 12% improvement over traditional CNN-based models. Additionally, the optimized architecture reduces computational overheads, ensuring faster processing suitable for real-time authentication systems. These findings highlight the superiority of hybrid deep learning techniques in biometric security by providing a quantifiable enhancement in both accuracy and efficiency. This research contributes to the advancement of secure, adaptive, and high-performance fingerprint authentication systems, bridging the gap between theoretical advancements and real-world applications.
Full article
(This article belongs to the Special Issue Using New Technologies in Cyber Security Solutions (2nd Edition))
►▼
Show Figures
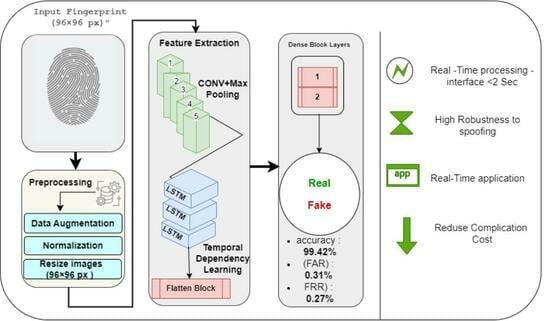
Graphical abstract
Open AccessArticle
Development and Evaluation of a Machine Learning Model for Predicting 30-Day Readmission in General Internal Medicine
by
Abdullah M. Al Alawi, Mariya Al Abdali, Al Zahraa Ahmed Al Mezeini, Thuraiya Al Rawahia, Eid Al Amri, Maisam Al Salmani, Zubaida Al-Falahi, Adhari Al Zaabi, Amira Al Aamri, Hatem Al Farhan and Juhaina Salim Al Maqbali
Computers 2025, 14(5), 177; https://doi.org/10.3390/computers14050177 - 5 May 2025
Abstract
►▼
Show Figures
Background/Objectives: Hospital readmissions within 30 days are a major challenge in general internal medicine (GIM), impacting patient outcomes and healthcare costs. This study aimed to develop and evaluate machine learning (ML) models for predicting 30-day readmissions in patients admitted under a GIM unit
[...] Read more.
Background/Objectives: Hospital readmissions within 30 days are a major challenge in general internal medicine (GIM), impacting patient outcomes and healthcare costs. This study aimed to develop and evaluate machine learning (ML) models for predicting 30-day readmissions in patients admitted under a GIM unit and to identify key predictors to guide targeted interventions. Methods: A prospective study was conducted on 443 patients admitted to the Unit of General Internal Medicine at Sultan Qaboos University Hospital between May and September 2023. Sixty-two variables were collected, including demographics, comorbidities, laboratory markers, vital signs, and medication data. Data preprocessing included handling missing values, standardizing continuous variables, and applying one-hot encoding to categorical variables. Four ML models—logistic regression, random forest, gradient boosting, and support vector machine (SVM)—were trained and evaluated. An ensemble model combining soft voting and weighted voting was developed to enhance performance, particularly recall. Results: The overall 30-day readmission rate was 14.2%. Among all models, logistic regression had the highest clinical relevance due to its balanced recall (70.6%) and area under the curve (AUC = 0.735). While random forest and SVM models showed higher precision, they had lower recall compared to logistic regression. The ensemble model improved recall to 70.6% through adjusted thresholds and model weighting, though precision declined. The most significant predictors of readmission included length of hospital stay, weight, age, number of medications, and abnormalities in liver enzymes. Conclusions: ML models, particularly ensemble approaches, can effectively predict 30-day readmissions in GIM patients. Tailored interventions using key predictors may help reduce readmission rates, although model calibration is essential to optimize performance trade-offs.
Full article
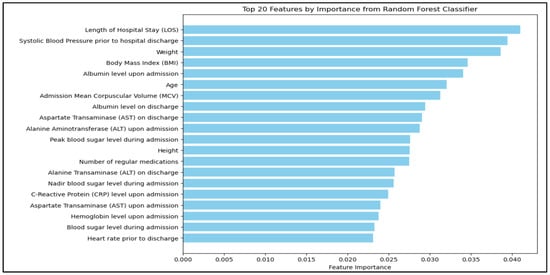
Figure 1
Open AccessArticle
Exploring Factors Influencing Students’ Continuance Intention to Use E-Learning System for Iraqi University Students
by
Ahmed Rashid Alkhuwaylidee
Computers 2025, 14(5), 176; https://doi.org/10.3390/computers14050176 - 5 May 2025
Abstract
In the past years, the education sector has suffered from repeated epidemics and their spread, and COVID-19 is a good example of this. Therefore, the search for other educational methods has become necessary. Therefore, e-learning is one of the best methods to replace
[...] Read more.
In the past years, the education sector has suffered from repeated epidemics and their spread, and COVID-19 is a good example of this. Therefore, the search for other educational methods has become necessary. Therefore, e-learning is one of the best methods to replace traditional education. In this study, we found it necessary to conduct a comprehensive st udy on the perceptions of Iraqi university students toward e-learning and the factors affecting its use by students’ interest in being used consistently to increase learning effectiveness and the influence of educational presentations. In this research, the Expectation−Confirmation Model was used as a framework, and SPSS v21 and AMOS v21 were used to analyze the questionnaire obtained from 360 valid samples. According to the findings, students’ perceptions of the usefulness of e-learning systems are influenced by factors such as system quality, content quality, and confirmation. In addition, the findings show that technical support has no effect on perceived usefulness. In addition, content quality, system quality, and technical support are three critical antecedents of confirmation. In addition, we found that satisfaction was positively affected by both confirmation and perceived usefulness. We also found that the continuance intention to use e-learning was positively affected by both satisfaction and perceived usefulness.
Full article
(This article belongs to the Special Issue Present and Future of E-Learning Technologies (2nd Edition))
►▼
Show Figures
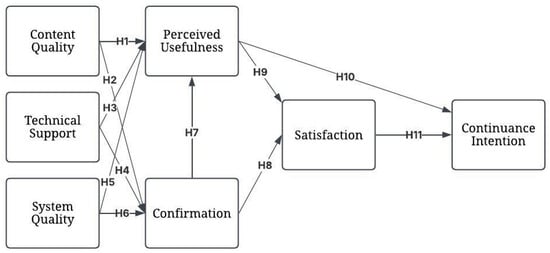
Figure 1
Open AccessArticle
Forecasting the Unseen: Enhancing Tsunami Occurrence Predictions with Machine-Learning-Driven Analytics
by
Snehal Satish, Hari Gonaygunta, Akhila Reddy Yadulla, Deepak Kumar, Mohan Harish Maturi, Karthik Meduri, Elyson De La Cruz, Geeta Sandeep Nadella and Guna Sekhar Sajja
Computers 2025, 14(5), 175; https://doi.org/10.3390/computers14050175 - 4 May 2025
Abstract
This research explores the improvement of tsunami occurrence forecasting with machine learning predictive models using earthquake-related data analytics. The primary goal is to develop a predictive framework that integrates a wide range of data sources, including seismic, geospatial, and ecological data, toward improving
[...] Read more.
This research explores the improvement of tsunami occurrence forecasting with machine learning predictive models using earthquake-related data analytics. The primary goal is to develop a predictive framework that integrates a wide range of data sources, including seismic, geospatial, and ecological data, toward improving the accuracy and lead times of tsunami occurrence predictions. The study employs machine learning methods, including Random Forest and Logistic Regression, for binary classification of tsunami events. Data collection is performed using a Kaggle dataset spanning 1995–2023, with preprocessing and exploratory analysis to identify critical patterns. The Random Forest model achieved superior performance with an accuracy of 0.90 and precision of 0.88 compared to Logistic Regression (accuracy: 0.89, precision: 0.87). These results underscore Random Forest’s effectiveness in handling imbalanced data. Challenges such as improving data quality and model interpretability are discussed, with recommendations for future improvements in real-time warning systems.
Full article
(This article belongs to the Special Issue Machine Learning and Statistical Learning with Applications 2025)
►▼
Show Figures
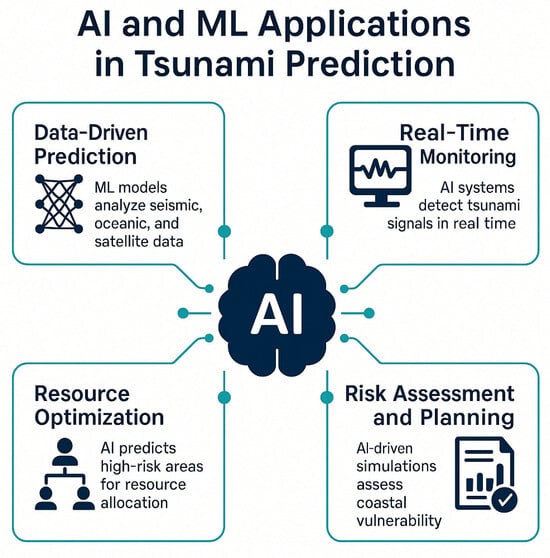
Figure 1
Open AccessArticle
Investigation of Multiple Hybrid Deep Learning Models for Accurate and Optimized Network Slicing
by
Ahmed Raoof Nasser and Omar Younis Alani
Computers 2025, 14(5), 174; https://doi.org/10.3390/computers14050174 - 2 May 2025
Abstract
In 5G wireless communication, network slicing is considered one of the key network elements, which aims to provide services with high availability, low latency, maximizing data throughput, and ultra-reliability and save network resources. Due to the exponential expansion of cellular networking in the
[...] Read more.
In 5G wireless communication, network slicing is considered one of the key network elements, which aims to provide services with high availability, low latency, maximizing data throughput, and ultra-reliability and save network resources. Due to the exponential expansion of cellular networking in the number of users along with the new applications, delivering the desired Quality of Service (QoS) requires an accurate and fast network slicing mechanism. In this paper, hybrid deep learning (DL) approaches are investigated using convolutional neural networks (CNNs), Long Short-Term Memory (LSTM), recurrent neural networks (RNNs), and Gated Recurrent Units (GRUs) to provide an accurate network slicing model. The proposed hybrid approaches are CNN-LSTM, CNN-RNN, and CNN-GRU, where a CNN is initially used for effective feature extraction and then LSTM, an RNN, and GRUs are utilized to achieve an accurate network slice classification. To optimize the model performance in terms of accuracy and model complexity, the hyperparameters of each algorithm are selected using the Bayesian optimization algorithm. The obtained results illustrate that the optimized hybrid CNN-GRU algorithm provides the best performance in terms of slicing accuracy (99.31%) and low model complexity.
Full article
(This article belongs to the Special Issue Distributed Computing Paradigms for the Internet of Things: Exploring Cloud, Edge, and Fog Solutions)
►▼
Show Figures
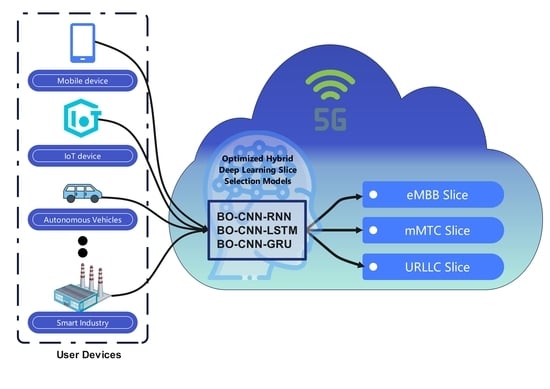
Graphical abstract
Open AccessArticle
Combining the Strengths of LLMs and Persuasive Technology to Combat Cyberhate
by
Malik Almaliki, Abdulqader M. Almars, Khulood O. Aljuhani and El-Sayed Atlam
Computers 2025, 14(5), 173; https://doi.org/10.3390/computers14050173 - 2 May 2025
Abstract
Cyberhate presents a multifaceted, context-sensitive challenge that existing detection methods often struggle to tackle effectively. Large language models (LLMs) exhibit considerable potential for improving cyberhate detection due to their advanced contextual understanding. However, detection alone is insufficient; it is crucial for software to
[...] Read more.
Cyberhate presents a multifaceted, context-sensitive challenge that existing detection methods often struggle to tackle effectively. Large language models (LLMs) exhibit considerable potential for improving cyberhate detection due to their advanced contextual understanding. However, detection alone is insufficient; it is crucial for software to also promote healthier user behaviors and empower individuals to actively confront the spread of cyberhate. This study investigates whether integrating large language models (LLMs) with persuasive technology (PT) can effectively detect cyberhate and encourage prosocial user behavior in digital spaces. Through an empirical study, we examine users’ perceptions of a self-monitoring persuasive strategy designed to reduce cyberhate. Specifically, the study introduces the Comment Analysis Feature to limit cyberhate spread, utilizing a prompt-based fine-tuning approach combined with LLMs. By framing users’ comments within the relevant context of cyberhate, the feature classifies input as either cyberhate or non-cyberhate and generates context-aware alternative statements when necessary to encourage more positive communication. A case study evaluated its real-world performance, examining user comments, detection accuracy, and the impact of alternative statements on user engagement and perception. The findings indicate that while most of the users (83%) found the suggestions clear and helpful, some resisted them, either because they felt the changes were irrelevant or misaligned with their intended expression (15%) or because they perceived them as a form of censorship (36%). However, a substantial number of users (40%) believed the interventions enhanced their language and overall commenting tone, with 68% suggesting they could have a positive long-term impact on reducing cyberhate. These insights highlight the potential of combining LLMs and PT to promote healthier online discourse while underscoring the need to address user concerns regarding relevance, intent, and freedom of expression.
Full article
(This article belongs to the Special Issue Recent Advances in Social Networks and Social Media)
►▼
Show Figures
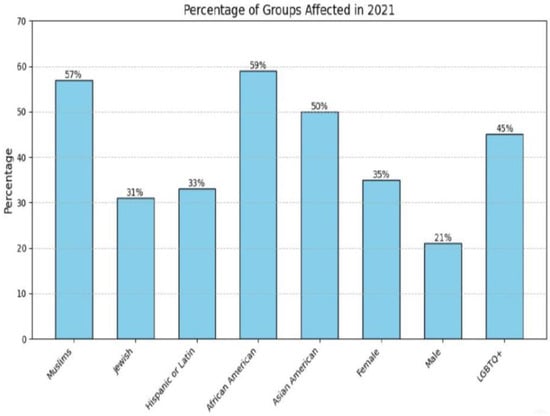
Figure 1
Open AccessArticle
A Framework for Domain-Specific Dataset Creation and Adaptation of Large Language Models
by
George Balaskas, Homer Papadopoulos, Dimitra Pappa, Quentin Loisel and Sebastien Chastin
Computers 2025, 14(5), 172; https://doi.org/10.3390/computers14050172 - 2 May 2025
Abstract
This paper introduces a novel framework for addressing domain adaptation challenges in large language models (LLMs), emphasising privacy-preserving synthetic data generation and efficient fine-tuning. The proposed framework employs a multi-stage approach that includes document ingestion, relevance assessment, and automated dataset creation. This process
[...] Read more.
This paper introduces a novel framework for addressing domain adaptation challenges in large language models (LLMs), emphasising privacy-preserving synthetic data generation and efficient fine-tuning. The proposed framework employs a multi-stage approach that includes document ingestion, relevance assessment, and automated dataset creation. This process reduces the need for extensive technical expertise while safeguarding data privacy. We evaluate the framework’s performance on domain-specific tasks in fields such as biobanking and public health, demonstrating that models fine-tuned using our method achieve results comparable to larger proprietary models. Crucially, these models maintain their general instruction-following capabilities, even when adapted to specialised domains, as shown through experiments with 7B and 8B parameter LLMs. Key components of the framework include continuous pre-training, supervised fine-tuning (SFT), and reinforcement learning methods such as direct preference optimisation (DPO), which together provide a flexible and configurable solution for deploying LLMs. The framework supports both local models and API-based solutions, making it scalable and accessible. By enabling privacy-preserving, domain-specific adaptation without requiring extensive expertise, this framework represents a significant step forward in the deployment of LLMs for specialised applications. The framework significantly lowers the barrier to domain adaptation for small- and medium-sized enterprises (SMEs), enabling them to utilise the power of LLMs without requiring extensive resources or technical expertise.
Full article
(This article belongs to the Special Issue Using New Technologies in Cyber Security Solutions (2nd Edition))
►▼
Show Figures
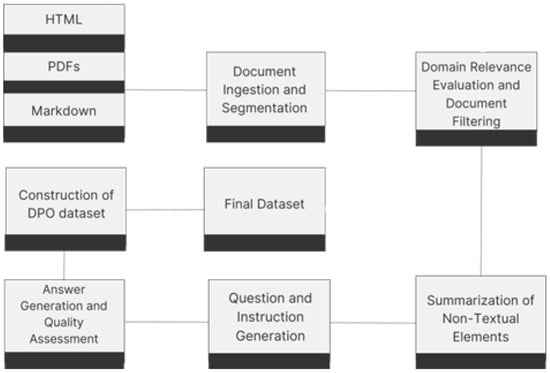
Figure 1
Open AccessArticle
ViX-MangoEFormer: An Enhanced Vision Transformer–EfficientFormer and Stacking Ensemble Approach for Mango Leaf Disease Recognition with Explainable Artificial Intelligence
by
Abdullah Al Noman, Amira Hossain, Anamul Sakib, Jesika Debnath, Hasib Fardin, Abdullah Al Sakib, Rezaul Haque, Md. Redwan Ahmed, Ahmed Wasif Reza and M. Ali Akber Dewan
Computers 2025, 14(5), 171; https://doi.org/10.3390/computers14050171 - 2 May 2025
Abstract
Mango productivity suffers greatly from leaf diseases, leading to economic and food security issues. Current visual inspection methods are slow and subjective. Previous Deep-Learning (DL) solutions have shown promise but suffer from imbalanced datasets, modest generalization, and limited interpretability. To address these challenges,
[...] Read more.
Mango productivity suffers greatly from leaf diseases, leading to economic and food security issues. Current visual inspection methods are slow and subjective. Previous Deep-Learning (DL) solutions have shown promise but suffer from imbalanced datasets, modest generalization, and limited interpretability. To address these challenges, this study introduces the ViX-MangoEFormer, which combines convolutional kernels and self-attention to effectively diagnose multiple mango leaf conditions in both balanced and imbalanced image sets. To benchmark against ViX-MangoEFormer, we developed a stacking ensemble model (MangoNet-Stack) that utilizes five transfer learning networks as base learners. All models were trained with Grad-CAM produced pixel-level explanations. In a combined dataset of 25,530 images, ViX-MangoEFormer achieved an F1 score of 99.78% and a Matthews Correlation Coefficient (MCC) of 99.34%. This performance consistently outperformed individual pre-trained models and MangoNet-Stack. Additionally, data augmentation has improved the performance of every architecture compared to its non-augmented version. Cross-domain tests on morphologically similar crop leaves confirmed strong generalization. Our findings validate the effectiveness of transformer attention and XAI in mango leaf disease detection. ViX-MangoEFormer is deployed as a web application that delivers real-time predictions, probability scores, and visual rationales. The system enables growers to respond quickly and enhances large-scale smart crop health monitoring.
Full article
(This article belongs to the Special Issue Deep Learning and Explainable Artificial Intelligence)
►▼
Show Figures
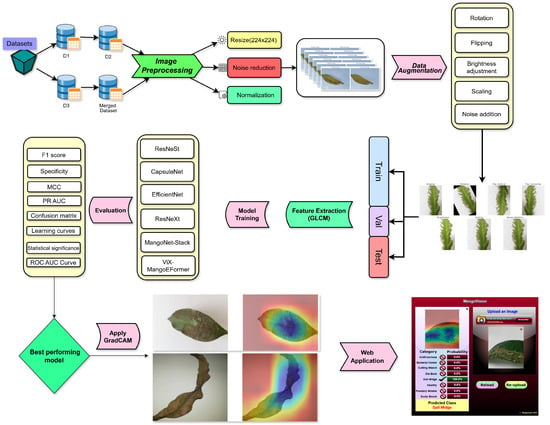
Figure 1
Open AccessArticle
Application of Graphics Processor Unit Computing Resources to Solution of Incompressible Fluid Dynamics Problems
by
Redha Benhadj-Djilali, Arturas Gulevskis and Konstantin Volkov
Computers 2025, 14(5), 170; https://doi.org/10.3390/computers14050170 - 1 May 2025
Abstract
►▼
Show Figures
The structure and memory organization of graphics processor units (GPUs) manufactured by NVIDIA and the use of CUDA programming technology to solve computational fluid dynamics (CFD) problems is reviewed and discussed. The potential of using a general-purpose GPU to solve fluid dynamics problems
[...] Read more.
The structure and memory organization of graphics processor units (GPUs) manufactured by NVIDIA and the use of CUDA programming technology to solve computational fluid dynamics (CFD) problems is reviewed and discussed. The potential of using a general-purpose GPU to solve fluid dynamics problems is examined. The code optimization with the utilization of various memory types is considered. Some CFD benchmark problems focused on simulation of viscous incompressible fluid flows are solved on GPUs. Consideration is given to the application of the finite volume method and projection method. Programming implementation of various components of the computational procedure, solution of Poisson equation for pressure and multigrid method to solve the system of algebraic equations, is provided. By using meshes of varying resolutions and different techniques for dividing up the input data into blocks, the speedup of the GPU solution is compared to the CPU approach.
Full article
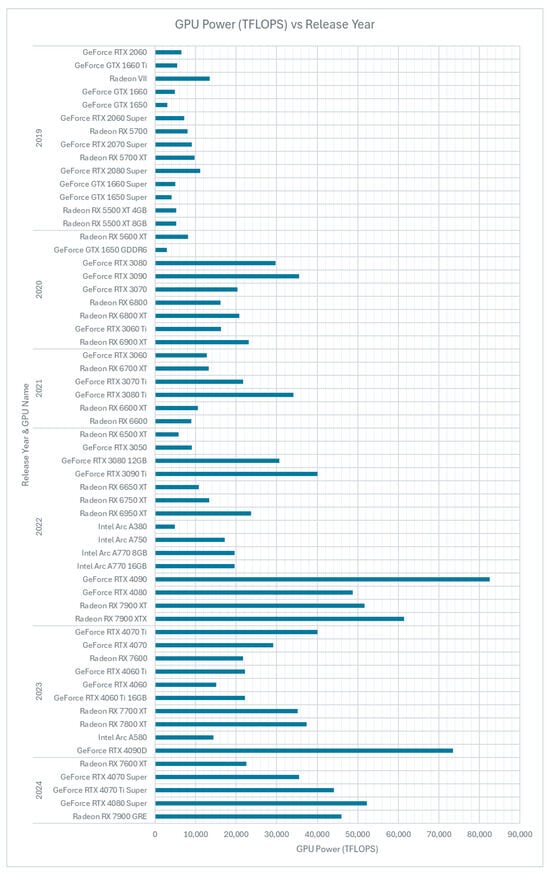
Figure 1
Open AccessArticle
Leveraging Technology to Break Barriers in Public Health for Students with Intellectual Disabilities
by
Georgia Iatraki, Tassos A. Mikropoulos, Panos Mallidis-Malessas and Carolina Santos
Computers 2025, 14(5), 169; https://doi.org/10.3390/computers14050169 - 1 May 2025
Abstract
A key goal of inclusive education is to enhance health literacy skills, empowering students with intellectual disabilities (IDs) to access critical information needed to navigate everyday challenges. The COVID-19 pandemic, for example, highlighted unique barriers to preparedness for people with IDs regarding social
[...] Read more.
A key goal of inclusive education is to enhance health literacy skills, empowering students with intellectual disabilities (IDs) to access critical information needed to navigate everyday challenges. The COVID-19 pandemic, for example, highlighted unique barriers to preparedness for people with IDs regarding social behavior and decision-making. This study aimed to examine students’ awareness and understanding of pandemic outbreaks. Using an inquiry-based approach supported by Digital Learning Objects (DLOs), the research assessed students’ knowledge and perceptions of viruses, modes of transmission, and preventive measures. An in-depth visual analysis within a single-subject research design demonstrated that interdisciplinary educational scenarios on infectious diseases can be effective for students with ID, especially when DLOs are integrated with targeted instructional techniques.
Full article
(This article belongs to the Special Issue Transformative Approaches in Education: Harnessing AI, Augmented Reality, and Virtual Reality for Innovative Teaching and Learning)
►▼
Show Figures
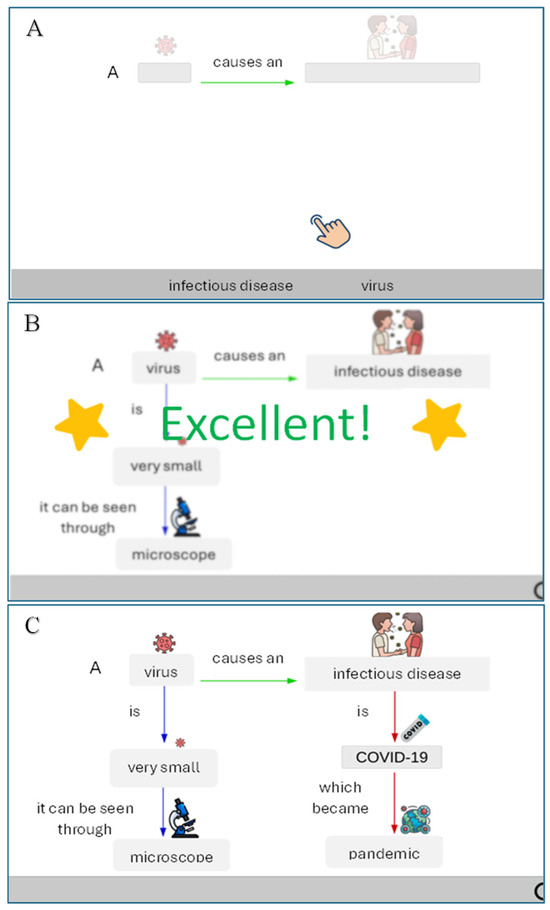
Figure 1
Highly Accessed Articles
Latest Books
E-Mail Alert
News
Topics
Topic in
Applied Sciences, ASI, Blockchains, Computers, MAKE, Software
Recent Advances in AI-Enhanced Software Engineering and Web Services
Topic Editors: Hai Wang, Zhe HouDeadline: 31 May 2025
Topic in
Applied Sciences, Computers, Electronics, Sensors, Virtual Worlds, IJGI
Simulations and Applications of Augmented and Virtual Reality, 2nd Edition
Topic Editors: Radu Comes, Dorin-Mircea Popovici, Calin Gheorghe Dan Neamtu, Jing-Jing FangDeadline: 20 June 2025
Topic in
Applied Sciences, Automation, Computers, Electronics, Sensors, JCP, Mathematics
Intelligent Optimization, Decision-Making and Privacy Preservation in Cyber–Physical Systems
Topic Editors: Lijuan Zha, Jinliang Liu, Jian LiuDeadline: 31 August 2025
Topic in
Animals, Computers, Information, J. Imaging, Veterinary Sciences
AI, Deep Learning, and Machine Learning in Veterinary Science Imaging
Topic Editors: Vitor Filipe, Lio Gonçalves, Mário GinjaDeadline: 31 October 2025

Conferences
Special Issues
Special Issue in
Computers
Future Trends in Computer Programming Education
Guest Editor: Stelios XinogalosDeadline: 31 May 2025
Special Issue in
Computers
Harnessing the Blockchain Technology in Unveiling Futuristic Applications
Guest Editors: Raman Singh, Shantanu PalDeadline: 15 June 2025
Special Issue in
Computers
Machine Learning Applications in Pattern Recognition
Guest Editor: Xiaochen LuDeadline: 30 June 2025
Special Issue in
Computers
When Natural Language Processing Meets Machine Learning—Opportunities, Challenges and Solutions
Guest Editors: Lu Bai, Huiru Zheng, Zhibao WangDeadline: 30 June 2025